Doc App - PCA Online For Free
Note: Integration described on this webpage may temporarily not be available.
0
Forms filled
0
Forms signed
0
Forms sent
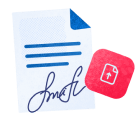
Upload your document to the PDF editor
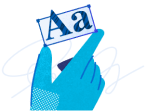
Type anywhere or sign your form
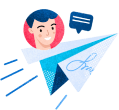
Print, email, fax, or export
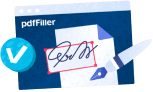
Try it right now! Edit pdf
Users trust to manage documents on pdfFiller platform
All-in-one PDF software
A single pill for all your PDF headaches. Edit, fill out, eSign, and share – on any device.
What our customers say about pdfFiller
See for yourself by reading reviews on the most popular resources:
Administrator in Construction
2019-05-31
Charlene B.
2017-11-14
needed a PDF filler software easy to use
easy to fill out lots of PDF forms without hassle
Easy to use. Can save forms for future use. Does not require any extra learning. Intuitive format makes easy for anyone to use.
Cost was a bit much, but decided the year membership was the best savings deal.
Have used it multiple times already in the first few months so I am glad I did not do a month to month membership.
Get a powerful PDF editor for your Mac or Windows PC
Install the desktop app to quickly edit PDFs, create fillable forms, and securely store your documents in the cloud.
Edit and manage PDFs from anywhere using your iOS or Android device
Install our mobile app and edit PDFs using an award-winning toolkit wherever you go.
Get a PDF editor in your Google Chrome browser
Install the pdfFiller extension for Google Chrome to fill out and edit PDFs straight from search results.
pdfFiller scores top ratings in multiple categories on G2
For pdfFiller’s FAQs
Below is a list of the most common customer questions. If you can’t find an answer to your question, please don’t hesitate to reach out to us.
What are the applications of PCA?
PCA is predominantly used as a dimensionality reduction technique in domains like facial recognition, computer vision and image compression. It is also used for finding patterns in data of high dimension in the field of finance, data mining, bioinformatics, psychology, etc.
What is PCA used for?
Principal component analysis (PCA) is a technique used to emphasize variation and bring out strong patterns in a dataset. It's often used to make data easy to explore and visualize.
What is the purpose of PCA?
PCA is mostly used as a tool in exploratory data analysis and for making predictive models. PCA is the simplest of the true eigenvector-based multivariate analyses. Often, its operation can be thought of as revealing the internal structure of the data in a way that best explains the variance in the data.
What is PCA in machine learning?
Principal Component Analysis (PCA) is a statistical procedure that uses an orthogonal transformation which converts a set of correlated variables to a set of uncorrelated variables. PCA is a most widely used tool in exploratory data analysis and in machine learning for predictive models.
What type of data should be used for PCA?
PCA works best on data set having 3 or higher dimensions. Because, with higher dimensions, it becomes increasingly difficult to make interpretations from the resultant cloud of data. PCA is applied on a data set with numeric variables.
How do you explain PCA?
Principal Component Analysis, or PCA, is a dimensionality-reduction method that is often used to reduce the dimensionality of large data sets, by transforming a large set of variables into a smaller one that still contains most of the information in the large set.
What is PCA ML?
Principal Component Analysis (PCA) is a statistical procedure that uses an orthogonal transformation which converts a set of correlated variables to a set of uncorrelated variables. PCA is a most widely used tool in exploratory data analysis and in machine learning for predictive models.
Is PCA a learning machine?
Principal Component Analysis (PCA) is an unsupervised, non-parametric statistical technique primarily used for dimensionality reduction in machine learning. Models also become more efficient as the reduced feature set boosts learning rates and diminishes computation costs by removing redundant features.
eSignature workflows made easy
Sign, send for signature, and track documents in real-time with signNow.
Easy to use, saves your signature, can merge
What do you dislike?
The saving document feature is a little slow
What problems are you solving with the product? What benefits have you realized?
Easy to fill PDFs without printing. Can fill as I he info and mAkes edits a breeze