We examine the characteristics of adversary traffic and the system mechanisms used to respond to them. We present algorithms for detecting adversarial traffic in a distributed system. Furthermore, we show how adversarial traffic can be detected through dynamic modeling, and by applying adversarial traffic detection and response using a novel machine learning framework. Finally, we identify the types of adversarial traffic that can impact the system. This work makes use of some advances in machine learning that have emerged in the past year and shows how adversaries can have real impacts on our infrastructure, while remaining invisible for the majority of users. Keywords: adversarial advertisements attacks, adversarial advertisement detection & response, adversarial ad networks, online advertising network, dynamic classification of adversarial advertising.
2. Abstract Adversarial advertising relies on actors that are motivated to generate misleading or deceptive ads (e.g. spammers, fraudsters, etc). While there have been several research papers demonstrating these kinds of attacks using web-based advertising systems (e.g. [6, 7, 8]), this research paper will focus on an entirely different class of threats: those that occur between the content of an advertisement and the machine-generated response to this advertisement. An attacker seeking to exploit the vulnerabilities presented here can craft an advertisement that will be perceived as highly desirable for human audiences, e.g. one that contains both attractive images, but also contains links that deliver malware from a remote server. These kinds of advertisements are called adversarial advertisements. We are concerned with detecting adversarial advertisements from within a machine-learning model, while ignoring the content of the advertisement itself. Thus, we construct machine-learned models for the following domains of adversarial advertisement: the content and format of individual advertisements, the content and format of the response that is generated upon receipt of an adversarial advertisement, and their similarity to advertisements without an adversarial result.
3. Requirements for Detection The detection of adversarial advertisements requires both training an adversarial network for the purposes of creating and propagating adversarial responses to adversarial advertisements, and then training a system using the adversarial network to evaluate the responses generated by the underlying model. These two tasks are, to some degree, complementary to each other. Since adversarial advertising occurs at the edge of the information system and is typically invisible to users, the most practical way to train a network for detection of adversarial advertisements (for both systems) appears to be to train an adversarial network at its source of control.

Get the free detecting adversarial advertisements in search result form
Show details
Detecting Adversarial Advertisements in the Wild D. Sculley Google, Inc. Matthew Eric Okay Google, Inc. Michael Poll Google, Inc. Sculley google.com Bridget Spitznagel Google, Inc. okay google.com
We are not affiliated with any brand or entity on this form
Get, Create, Make and Sign
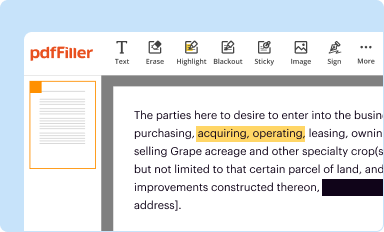
Edit your detecting adversarial advertisements in form online
Type text, complete fillable fields, insert images, highlight or blackout data for discretion, add comments, and more.
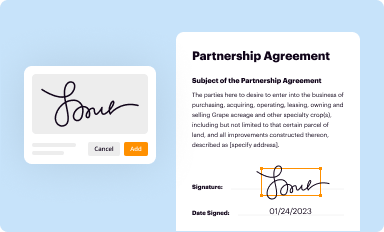
Add your legally-binding signature
Draw or type your signature, upload a signature image, or capture it with your digital camera.
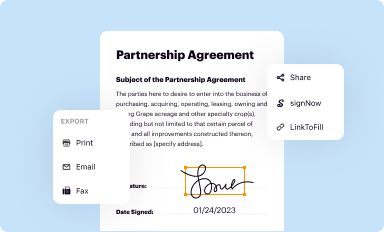
Share your form instantly
Email, fax, or share your detecting adversarial advertisements in form via URL. You can also download, print, or export forms to your preferred cloud storage service.
How to edit detecting adversarial advertisements in online
Here are the steps you need to follow to get started with our professional PDF editor:
1
Create an account. Begin by choosing Start Free Trial and, if you are a new user, establish a profile.
2
Simply add a document. Select Add New from your Dashboard and import a file into the system by uploading it from your device or importing it via the cloud, online, or internal mail. Then click Begin editing.
3
Edit detecting adversarial advertisements in. Rearrange and rotate pages, add and edit text, and use additional tools. To save changes and return to your Dashboard, click Done. The Documents tab allows you to merge, divide, lock, or unlock files.
4
Get your file. Select the name of your file in the docs list and choose your preferred exporting method. You can download it as a PDF, save it in another format, send it by email, or transfer it to the cloud.
It's easier to work with documents with pdfFiller than you could have believed. You can sign up for an account to see for yourself.
Fill form : Try Risk Free
For pdfFiller’s FAQs
Below is a list of the most common customer questions. If you can’t find an answer to your question, please don’t hesitate to reach out to us.
What is detecting adversarial advertisements in?
Detecting adversarial advertisements is a process of identifying and identifying malicious or misleading advertisements that aim to deceive or defraud users.
Who is required to file detecting adversarial advertisements in?
The responsibility for filing detecting adversarial advertisements lies with the advertising platforms, such as social media platforms or search engines, who display or distribute these advertisements.
How to fill out detecting adversarial advertisements in?
The process of filling out detecting adversarial advertisements involves implementing algorithms or systems that can analyze and classify advertisements based on various criteria, such as content, context, and user feedback. These systems can be developed using machine learning techniques and trained using labeled datasets.
What is the purpose of detecting adversarial advertisements in?
The purpose of detecting adversarial advertisements is to protect users from harmful or fraudulent advertising practices. By identifying and removing these advertisements, the integrity and trustworthiness of the advertising ecosystem can be maintained.
What information must be reported on detecting adversarial advertisements in?
The information that should be reported on detecting adversarial advertisements may include details about the detected advertisements, such as the type of malicious behavior, the advertising platform where it was found, and any associated user feedback or complaints.
When is the deadline to file detecting adversarial advertisements in in 2023?
The specific deadline for filing detecting adversarial advertisements in 2023 may vary depending on the regulations or policies set by the advertising platforms or relevant authorities. It is advisable to consult the specific guidelines provided by the respective platforms or regulatory bodies.
What is the penalty for the late filing of detecting adversarial advertisements in?
The penalties for the late filing of detecting adversarial advertisements may also vary depending on the regulations and policies established by the advertising platforms or regulatory bodies. The penalties can range from warnings and fines to potential suspension or revocation of advertising privileges.
How can I modify detecting adversarial advertisements in without leaving Google Drive?
By combining pdfFiller with Google Docs, you can generate fillable forms directly in Google Drive. No need to leave Google Drive to make edits or sign documents, including detecting adversarial advertisements in. Use pdfFiller's features in Google Drive to handle documents on any internet-connected device.
Can I sign the detecting adversarial advertisements in electronically in Chrome?
Yes. By adding the solution to your Chrome browser, you may use pdfFiller to eSign documents while also enjoying all of the PDF editor's capabilities in one spot. Create a legally enforceable eSignature by sketching, typing, or uploading a photo of your handwritten signature using the extension. Whatever option you select, you'll be able to eSign your detecting adversarial advertisements in in seconds.
Can I edit detecting adversarial advertisements in on an Android device?
With the pdfFiller Android app, you can edit, sign, and share detecting adversarial advertisements in on your mobile device from any place. All you need is an internet connection to do this. Keep your documents in order from anywhere with the help of the app!
Fill out your detecting adversarial advertisements in online with pdfFiller!
pdfFiller is an end-to-end solution for managing, creating, and editing documents and forms in the cloud. Save time and hassle by preparing your tax forms online.

Not the form you were looking for?
Keywords
Related Forms
If you believe that this page should be taken down, please follow our DMCA take down process
here
.